Contrasting two bottlenecks in the ACT-R architecture
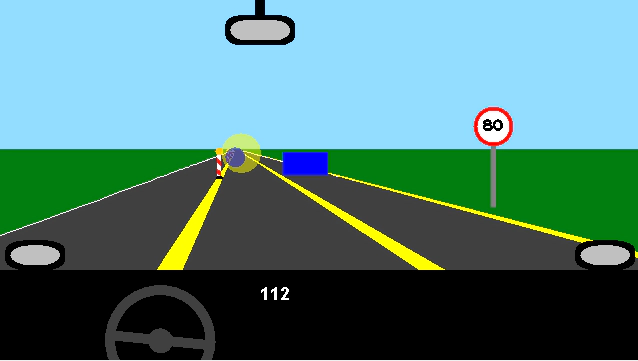
Project Description
This work was motivated by the findings of Jakob Scheunemann, Anirudh Unni and Jochem Rieger, who found interactions between two cognitive concepts while driving. These interactions have been attributed to a central bottleneck or to the so-called problem-state bottleneck, related to working memory usage. We developed two different cognitive models in the cognitive architecture ACT-R, which implement the two different bottlenecks. The models performed a highway driving task, during which we varied visuospatial attention and working memory load. To evaluate the models, we conducted an experiment with human participants and compared the behavioral data to the model’s behavior.